Application of ARIMA and 2D-CNNs Using Recurrence Plots for Medium-Term Load Forecasting
ID:285
Submission ID:81 View Protection:ATTENDEE
Updated Time:2021-12-10 13:51:22
Hits:672
Poster Presentation
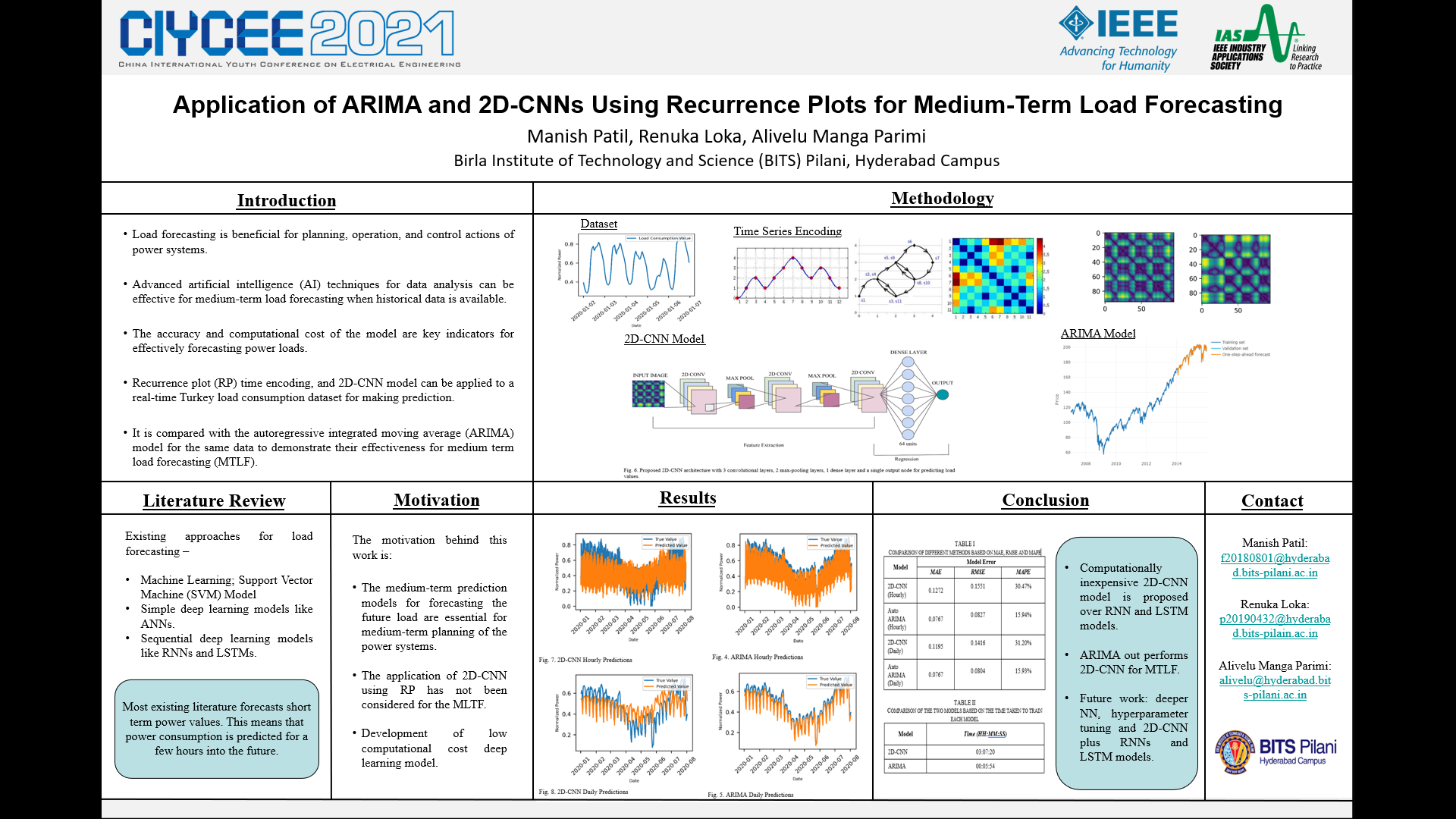
Start Time:2021-12-17 14:35 (Asia/Shanghai)
Duration:5min
Session:[Z] Poster Session » [Z6] Poster Session 6: AI-driven technology
Video
No Permission
Presentation File
Attachment File
Tips: The file permissions under this presentation are only for participants. You have not logged in yet and cannot view it temporarily.
Abstract
Load forecasting is beneficial for planning, operation, and control actions of power systems. Historical documented real-time load data can be utilized to predict the future load on power systems. In this regard the advanced artificial intelligence (AI) techniques for data analysis can be effective for medium-term load forecasting. The accuracy and computational cost of the model are key indicators for effectively forecasting power loads. In this paper, a recurrence plot (RP) time encoding, and 2D-CNN model is applied to a real-time Turkey load consumption dataset for making prediction and is compared with the autoregressive integrated moving average (ARIMA) model for the same data to demonstrate their effectiveness for medium term load forecasting.
Keywords
2D Convolutional Neural Networks,ARIMA,Power Load Forecasting,Recurrence Plots
Speaker
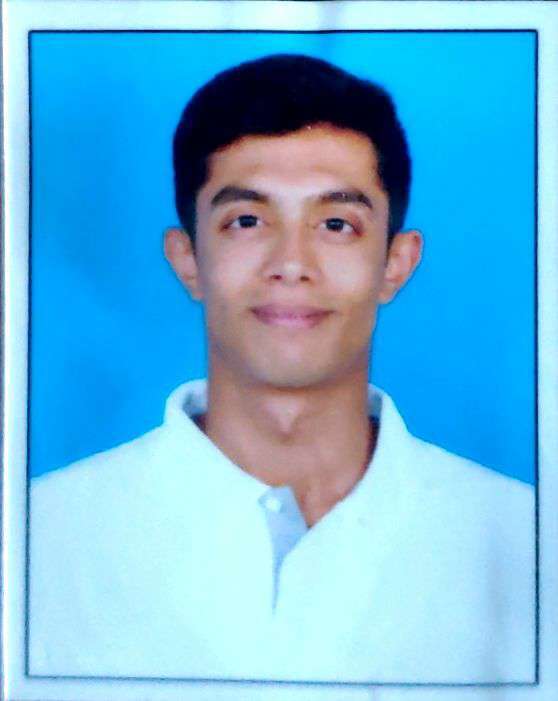
Comment submit