Few-Shot Transfer Learning with Attention Mechanism for High-Voltage Circuit Breaker fault diagnosis
ID:283
Submission ID:96 View Protection:ATTENDEE
Updated Time:2021-12-03 10:56:59 Hits:652
Oral Presentation
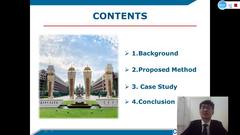
Start Time:2021-12-15 15:15 (Asia/Shanghai)
Duration:15min
Session:[F] AI-driven technology » [F1] Session 6
Video
No Permission
Presentation File
Tips: The file permissions under this presentation are only for participants. You have not logged in yet and cannot view it temporarily.
Abstract
Data-driven artificial intelligence methods, especially convolutional neural networks (CNNs), have achieved excellent performance in high-voltage circuit breaker mechanical fault diagnosis due to their powerful feature extraction and classification capabilities. However, CNN relies heavily on massive data. When the amount of data decreases, the fault diagnosis performance drops severely. To solve the above problems, this paper proposes a few-shot transfer learning (FSTL) with attention mechanism to realize the mechanical fault diagnosis of high-voltage circuit breakers. First, a one-dimensional CNN (1DCNN) with attention mechanism (AM) is used to extract the mechanical fault features of high-voltage circuit breakers. The introduction of the AM makes CNN pay more attention to the interesting part of the fault signal to extract effective key features. Then, domain adaptive transfer learning (DATL) is used to realize the deployment and application of 1DCNN constructed under a large amount of low-voltage level data to small samples of ultra-high voltage (UHV), so as to realize reliable diagnosis of UHV circuit breakers in small samples. The proposed DATL can consider the marginal distribution and conditional distribution of the two data at the same time to achieve better feature matching. Experimental results show that the FSTL proposed can achieve highly accurate and robust fault diagnosis of high-voltage circuit breakers with few-shot on site. Compared with the traditional method, the method proposed in this paper is obvious and provides a reliable reference for the diagnosis of high-voltage circuit breakers.
Keywords
domain adaptive transfer learning, fault diagnosis, few-shot, high-voltage circuit breakers, one-dimensional convolutional neural network
Speaker

Comment submit