High Impedance Fault Diagnosis Method Based on Conditional Wasserstein Generative Adversarial Network
ID:262
Submission ID:359 View Protection:ATTENDEE
Updated Time:2021-12-10 18:48:03
Hits:673
Oral Presentation
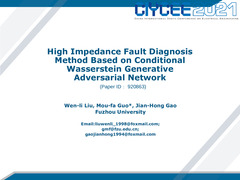
Start Time:2021-12-15 17:00 (Asia/Shanghai)
Duration:15min
Session:[F] AI-driven technology » [F2] Session 12
Video
No Permission
Presentation File
Tips: The file permissions under this presentation are only for participants. You have not logged in yet and cannot view it temporarily.
Abstract
Data-driven fault diagnosis of high impedance fault (HIF) has received increasing attention and achieved fruitful results. However, HIF data is difficult to obtain in engineering. Furthermore, there exists an imbalance between the fault data and non-fault data, making data-driven methods hard to detect HIFs reliably under the small imbalanced sample condition. To solve this problem, this paper proposes a novel HIF diagnosis method based on conditional Wasserstein generative adversarial network (WCGAN). By adversarial training, the generator can generate sufficient labeled zero-sequence current signals, which can be used as training data to expand the limited training set and achieve the balanced distribution of the samples. In addition, the Wasserstein distance was introduced to improve the loss function. Experimental results indicate that the proposed method can generate high-quality samples and achieve a high accuracy rate of fault detection in the case of small imbalanced samples.
Keywords
high impedance fault, fault diagnosis, small imbalanced sample, generative adversarial network, data augmentation
Speaker

Comment submit