An imputation method based on dummy variable and unsupervised learning for electricity consumption data with missing values
ID:293
Submission ID:19 View Protection:ATTENDEE
Updated Time:2021-12-03 10:58:01 Hits:400
Oral Presentation
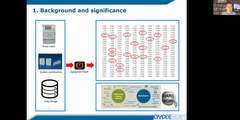
Start Time:2021-12-15 14:30 (Asia/Shanghai)
Duration:15min
Session:[F] AI-driven technology » [F1] Session 6
Video
No Permission
Presentation File
Tips: The file permissions under this presentation are only for participants. You have not logged in yet and cannot view it temporarily.
Abstract
Due to the fault of smart meters, system maintenance, data storage, and other reasons, part of the electricity consumption data is missing, which brings some difficulties to the behavior analysis of electricity consumption data. And deleting the missing samples will cause a loss of electricity information and detection model accuracy. Aiming at these problems, this paper proposes an imputation method based on dummy variable and unsupervised learning, and impute the missing values of electricity consumption data without affecting the quality of data. First, the dummy variable considers the missing information mode in the users’ electricity consumption and saves the important missing information. Combined with the deep learning model to learn the potential feature representation of electricity consumption data, and effectively realizes the complex relationship between variables through nonlinear transformation. The generated network and discriminant network are employed to generate the missing values and discriminate the imputation values to reduce the model error, thus providing a great imputation model with missing information to estimate the missing values of electricity consumption data. The root mean square error (RMSE) of different imputation models based on different datasets and different missing rates verifies that the proposed missing imputation model can more accurately and efficiently impute the missing values of electricity consumption data.
Keywords
electricity consumption, imputation method, dummy variable, unsupervised learning, missing values
Speaker

Comment submit