An Open-Source Toolbox with Classical Classifiers for Electricity Theft Detection
ID:179
Submission ID:37 View Protection:ATTENDEE
Updated Time:2021-12-05 05:51:06
Hits:396
Oral Presentation
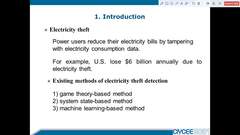
Start Time:2021-12-16 10:00 (Asia/Shanghai)
Duration:15min
Session:[H] Other topics in Electrical Engineering » [H1] Session 18
Video
No Permission
Presentation File
Tips: The file permissions under this presentation are only for participants. You have not logged in yet and cannot view it temporarily.
Abstract
Recently, there is increasing interest in detecting electricity thieves for economic benefits for power companies, and many works aim to improve the accuracy of electricity theft detection. Nevertheless, a core obstacle that currently hinders the direct comparison of classifiers for electricity theft detection is the lack of a standard and public dataset, since fraudulent power load profiles are usually difficult to collect for various reasons, including cost, cumber, and confidentiality. Therefore, this paper presents an open-source toolbox, which generates different kinds of fraudulent power load profiles from attack models, and integrates classical classifiers (e.g., support vector machine, multi-layer perceptron, convolutional neural network, long short-term memory, bidirectional long short-term memory) with different performance as baselines for the comparison with new algorithms. Users can easily generate datasets and modify parameters of classical classifiers guided by user friendly interactive interfaces. The codes, toolbox, and user manuals are available online and it is free to use and extend them.
Keywords
Electricity theft detection, toolbox, attack models, power load, classifier
Speaker

Comment submit